The Sum of Squared Residuals (SSR), also known as the Sum of Squared Errors (SSE), can be derived from the standard error of the estimate in the context of a regression model. Here’s how you can do it:
Understanding the Concepts
- SSR (Sum of Squared Residuals): This is the sum of the squares of the differences between the observed values and the predicted values..
- Standard Error of the Estimate: This is a measure of the accuracy of predictions made with a regression line. It’s essentially the standard deviation of the residuals (or prediction errors).

Relationship between SSR and Standard Error
The standard error of the estimate (SE) is related to the SSR as follows:
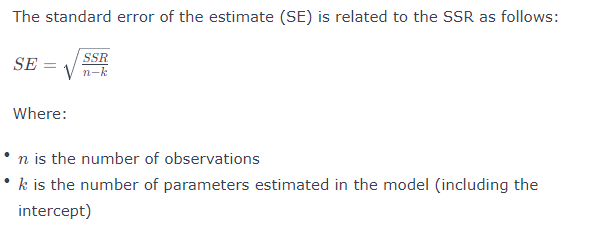
Calculating SSR from Standard Error
Given the standard error (SE), you can rearrange the formula to solve for SSR:

This formula allows you to calculate the SSR if you know the standard error of the regression and the number of observations and parameters in your model.
Example

This means the sum of squared residuals for your model is 392.